Overview
GPU Accelerated LLM Langchain AI Agent
About Advantech Container Catalog
Advantech Container Catalog is a comprehensive collection of ready-to-use, containerized software packages designed to accelerate the development and deployment of Edge AI applications. By offering pre-integrated solutions optimized for embedded hardware, it simplifies the challenges often faced with software and hardware compatibility, especially in GPU/NPU-accelerated environments.
Key benefits of the Container Catalog include:
Feature / Benefit | Description |
---|---|
Accelerated Edge AI Development | Ready-to-use containerized solutions for fast prototyping and deployment |
Hardware Compatibility Solved | Eliminates embedded hardware and AI software package incompatibility |
GPU/NPU Access Ready | Supports passthrough for efficient hardware acceleration |
Model Conversion & Optimization | Built-in AI model quantization and format conversion support |
Optimized for CV & LLM Applications | Pre-optimized containers for computer vision and large language models |
Scalable Device Management | Supports large-scale IoT deployments via EdgeSync, Kubernetes, etc. |
Lower Entry Barrier for Developers | High-level language (Python, C#, etc.) support enables easier development |
Developer Accessibility | Junior engineers can build embedded AI applications more easily |
Increased Customer Stickiness | Simplified tools lead to higher adoption and retention |
Open Ecosystem | 3rd-party developers can integrate new apps to expand the platform |
Container Overview
Turnkey LLM Edge AI Container, supported by variety of Advantech GPU accelerated hardwares & Containerized development environment with built-in GPU passthrough optimized for LLM /Agentic AI applications.
This Edge AI Container provides a modular, middleware-powered AI chat solution built for Advantech optimized hardware platforms. This stack uses Ollama with LLAMA 3.2 1b Model to serve LLMs, a FastAPI-based LangChain service for middleware logic, and OpenWebUI as the user interface.
This architecture enables tool-augmented reasoning, multi-turn memory, and custom LLM workflows using LangChain Agents.
User Benefits:
-
Plug-and-play LLM Container on Edge: supported by variety of Advantech AI-powered hardwares & Containerized environment with GPU passthrough optimized for LLM /Agentic AI applications.
-
Reduce Model Compilation Effort: Standarized model conversion and quantization workflow for users on Advantech optimized hardware platforms.
-
Flexible Harware Options: Select AI-powered hardware from Advantech’s verified list to best match your Edge AI application requirements—fully supported and optimized for this container.
Container Demo
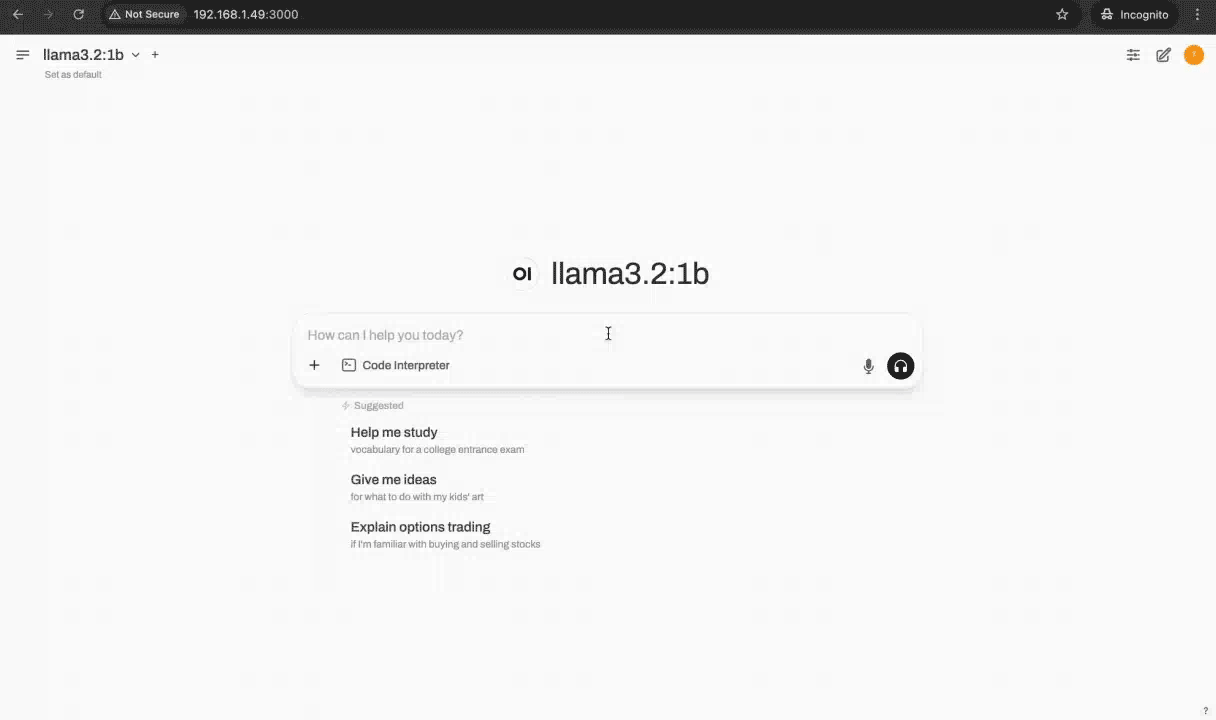
Key Features
Feature | Content |
---|---|
LLAMA 3.2 1b Inference | Efficient on-device LLM deployment via Ollama, optimized for edge environments with minimal memory usage and high-performance inference |
Integrated OpenWebUI | Clean, user-friendly frontend for interacting with LLMs via chat interface |
Custom Model Creation | Build or fine-tune your own variants with ollama create |
REST API Access | Interact with models via a simple local HTTP API |
Flexible Parameters | Tune inference with temperature, top_k, repeat_penalty, etc |
Modelfile Customization | Define model behavior and parameters using a Docker-like file |
Prompt Templates | Automatically format conversations using common structures like chatml or llama |
LangChain Agent Integration | Enables tool-augmented reasoning, structured prompt handling, and advanced control flow logic for enhanced interactions |
LangChain Memory Support | Multi-turn chat via ConversationBufferMemory for contextual understanding |
FastAPI Middleware | Lightweight interface between OpenWebUI and LangChain |
Complete AI Framework Stack | PyTorch, TensorFlow, ONNX Runtime |
Industrial Vision Support | Accelerated OpenCVpipelines |
Edge AI Capabilities | Support for computer vision, LLMs, and time-series analysis |
Performance Optimized | Tuned specifically for Advantech EPC-R7300 and more devices |
Host Device Prerequisites
Item | Specification |
---|---|
Compatible Hardware | Optimzied and GPU accelerated Advantech devices - refer to Compatible hardware |
Host OS | Ubuntu 20.04 |
Required Software packages | Details refer to Advantech EdgeSync Container Repository |
Software Installation | Host Software Package Installation |
Container Environment Overview
Architecture
User → OpenWebUI → FastAPI (LangChain Agent) → Ollama Inference
Software Components on Container Image
Component | Version | Description |
---|---|---|
PyTorch | 2.0.0+nv23.02 | Deep learning framework |
TensorFlow | 2.12.0+nv23.05 | Machine learning framework |
ONNX Runtime | 1.16.3 | Cross-platform inference engine |
OpenCV | 4.5.0 | Computer vision library with GPU Toolkit |
GStreamer | 1.16.2 | Multimedia framework |
Model Information
Item | Description |
---|---|
Model source | Ollama Model (llama3.2:1b) |
Model architecture | llama |
Model quantization | Q8_0 |
Ollama command | ollama pull llama3.2:1b |
Number of Parameters | ~1.24 B |
Model size | ~1.3 GB |
Default context size (governed by Ollama in this image) | 2048 |
Language Model Recommendation & Performace Reference
Model Family | Versions | Memory Requirements | Performance Notes |
---|---|---|---|
DeepSeek R1 | 1.5B | ~1 GB | ~15-17 tokens/sec in Q4_K_M |
Llama 3.2 | 1B | ~1 GB | ~17-20 tokens/sec in Q8_0 |
DeepSeek Coder | Mini (1.3B), Light (1.5B) | 2-3 GB | |
TinyLlama | 1.1B | 2 GB | |
Phi | Phi-1.5 (1.3B), Phi-2 (2.7B) | 1.5-3 GB | |
Llama 2 | 7B (Quantized to 4-bit) | 3-4 GB | |
Mistral | 7B (Quantized to 4-bit) | 3-4 GB |
*The performance benchmarking is run on Advantech EPC-R7300.
Ollama Integration
This container leverages Ollama as the local inference engine to serve LLMs efficiently on systems. Ollama provides a lightweight and container-friendly API layer for running language models like LLAMA3.2-1b
without requiring cloud-based services.
Key Highlights:
- Local model inference via Ollama API (
http://localhost:11434/v1
) - Supports streaming output for chat-based UIs like OpenWebUI
- Works with quantized
.gguf
models optimized for edge hardware
Container Quick Start Guide
For container quick start, including docker-compose file, and more, please refer to Advantech GPU Accelerated LLM Langchain AI Agent Repository